Artificial-intelligence active flow control for reductIon of transport emissions
ACCREDITATION will develop AI flow-control tools for the drag reduction of road transport vehicles.Artificial-intelligence active flow control for reductIon of transport emissions
ACCREDITATION will develop AI flow-control tools for the drag reduction of road transport vehicles.Project Summary
Road transport is a significant source of greenhouse gas emissions, with a remarkable environmental impact. Truck drag reduction is a key player for next-generation low-impact ground transportation, independent of advances in motorization. It is clear that closed-loop control can achieve better performances than open-loop control for aerodynamic flows. The advances in machine-learning methods pave the way for new opportunities in this field.
The control laws obtained with machine-learning methods are often difficult to interpret, thus rendering more complex their generalization and applicability. Furthermore, the demonstration of their capabilities in experimental conditions is still at its infancy. The objective of ACCREDITATION is to develop and test a new concept for closed-loop active flow control for heavy vehicles based on artificial intelligence, with the aim of reducing drag and, consequently, emissions and environmental impact of ground transportation.
The objective of ACCREDITATION will be reached by answering the following research questions:
Which flow sensing and actuation strategies are more suitable for heavy ground vehicles?
To this purpose, we will explore the use of pressure sensors and we will minimize their number with sparse-sensor-placement tools. We will also select the most suitable actuators among pulsed jet and plasma actuators.
Can we develop efficient and interpretable techniques for control law identification?
We will train open-loop control strategies, obtaining effective drag and lateral force reduction. We will interpret control laws making use of dimensionality reduction techniques such as manifold learning.
Can these control laws be implemented in an experimental environment?
All the results will be then validated by attempting closed-loop control in the wind tunnel.
ACCREDITATION in a nutshell
Title: Artificial-intelligence active flow control for reductIon of transport emissions.
Goal: to develop and test a new concept for closed-loop active flow control for heavy
vehicles based on artificial intelligence, with the aim of reduction of drag and, consequently, of emissions and environmental impact of ground transportation.
Duration: 34 months (01/12/2022 – 30/09/2025)
Budget: 187.910,00 €
Grant No TED2021-131453B-I00), funded by MCIN/AEI/ 10.13039/501100011033 and by the “European Union NextGenerationEU/PRTR

Team
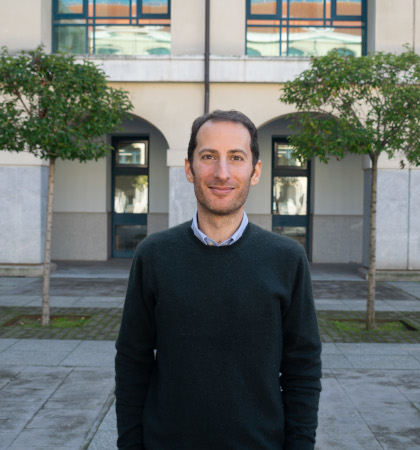
Andrea Ianiro
Principal Investigator
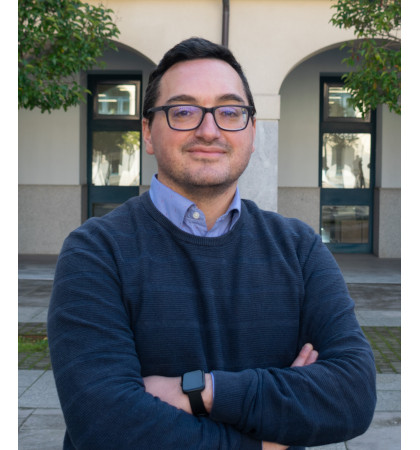
Stefano Discetti
Principal Investigator
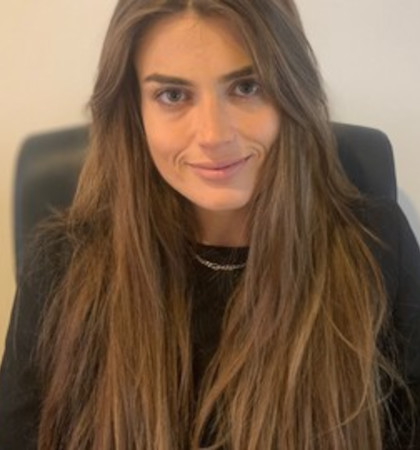
Andrea Meilán Vila
Associate Professor
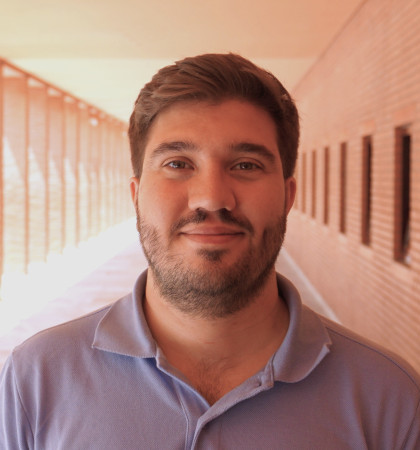
Carlos Sanmiguel Vila
Científico Titular
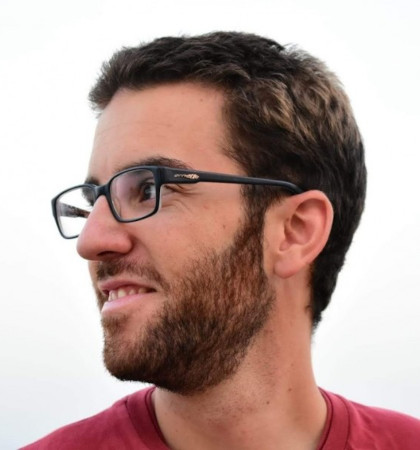
Alberto Solera Rico
PhD student
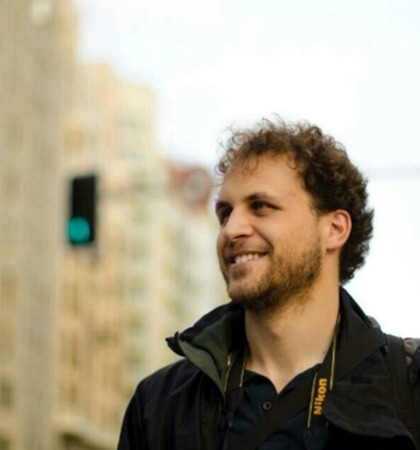
Miguel Ángel Gómez Lopez
PhD student
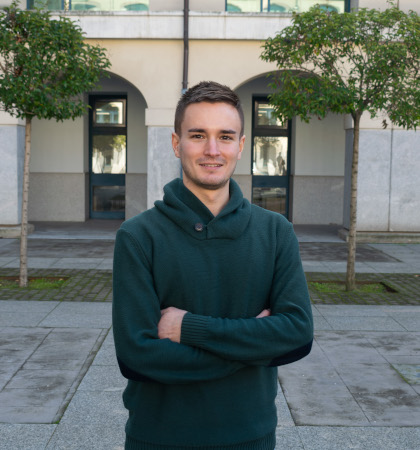
Antonio Cuéllar Martín
PhD student
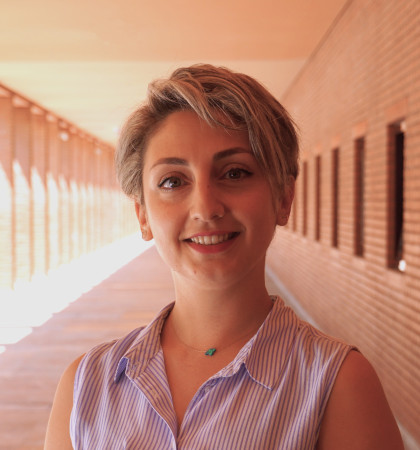
Firoozeh Foroozan
PhD student
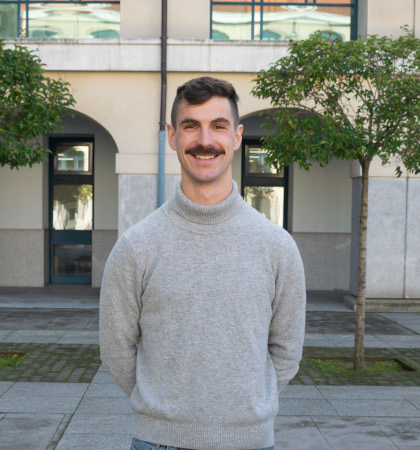
Lucas Scheeberger Carles
PhD student
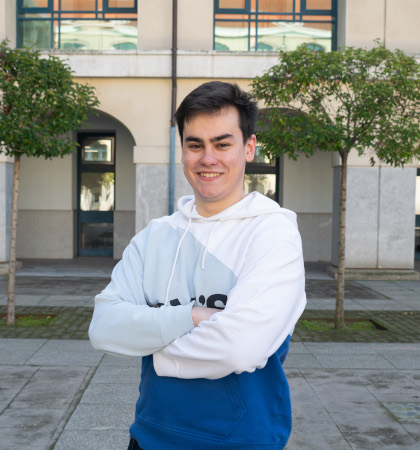
Isaac Robledo
Research Assistant
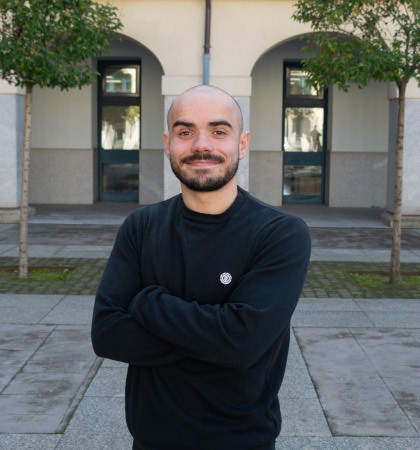
Juan Alfaro Moreno
Research Assistant
Results
Journal Papers
L. Marra, G.Y. Cornejo Maceda, A. Meilán-Vila, V. Guerrero, S. Rashwan, B.R. Noack, S. Discetti, A. Ianiro, “Actuation manifold from snapshot data”, Journal of Fluid Mechanics, 996:A26, 2024
Conference Contributions
L. Marra, A. Meilán-Vila, S. Discetti, “Drag reduction with self-tuning Model Predictive Control”, European Drag Reduction and Flow Control Meeting (EDRFCM) 2024, Torino, September 10-13, 2024.
L. Schneeberger, A. Ianiro, S. Discetti, “Plasma actuators for drag reduction on heavy ground vehicles”, European Drag Reduction and Flow Control Meeting (EDRFCM) 2024, Torino, September 10-13, 2024.
A. Solera, C. Sanmiguel, S. Discetti, “LSTM-based predictive modelling for active flow control of a wake”, European Drag Reduction and Flow Control Meeting (EDRFCM) 2024, Torino, September 10-13, 2024.
MSc and PhD Theses
A. Cuéllar, “AI-based sensing of turbulent wall-bounded flows”. (December 2024)
L. Schneeberger, “Machine-learning framework for active flow control around heavy ground vehicles” (expected defence October 2026)
L. Marra, “Model Predictive Control applied to turbulent flows” (expected defense June 2026)
A. Solera, “Investigation of flow control methods using data-driven algorithms”
Outreach Activities
L. Schneeberger, R. Moreno, “¿Fiat o Ferrari? Un experimento aerodinámico en vivo”, Madrid Science and Innovation Week 2024, Madrid, November 15
Open Science (datasets and codes)
L. Marra, G.Y. Cornejo Maceda, A. Meilán-Vila, V. Guerrero, S. Rashwan, B.R. Noack, S. Discetti, A. Ianiro, “Actuation manifold from snapshot data” (2024)
Data Set: https://zenodo.org/records/12802192
Github Codes: https://github.com/Lmarra1/Actuation-manifold-from-snapshot-data